Data traceability-based large-scale discrete feature mining method
A large-scale, discrete technology, applied in data mining, database models, multi-dimensional databases, etc., can solve problems such as loss of model iteration efficiency, inability to directly use production models, and large differences in architecture, and achieve the unification and development of offline data synchronization mechanisms. The effect of low maintenance cost and high model production efficiency
- Summary
- Abstract
- Description
- Claims
- Application Information
AI Technical Summary
Problems solved by technology
Method used
Image
Examples
Embodiment 1
[0021] A large-scale discrete feature mining method with traceable data. The same feature calculation lib is used for online requests and offline research. The raw data snapshots used for online feature calculation are fully saved in the cache to ensure the data used in offline research. The data is consistent with the data used online at that time. When there are new ideas for feature mining and new features need to be mined from the previous data, you only need to update the feature calculation lib, and use more data according to the large-scale discrete feature mining architecture. Sample making model.
[0022] First of all, it is necessary to ensure the separation of data acquisition and data calculation. The input of feature calculation lib is characterized by the output of original data. The cache can be realized by using different storage media (such as: mongo, redis, etc.) according to the needs. The data warehouse can be built based on the hadoop system (including: hd...
Embodiment 2
[0024] For a preferred structure of Embodiment 1, the large-scale discrete feature mining framework includes an offline system and an online system, the offline system is composed of a data warehouse, an offline feature mining system, and a model offline training system, and the offline feature mining system is passed through Load feature calculation lib to mine new features from the data warehouse, and the model offline training system uses new features to carry out model training; the online system is divided into three layers, a business layer, a feature layer, and a data storage layer. The business layer includes a business system, Risk control decision-making system, online estimation system, the business system sends the basic information of the order (including: order id, mobile phone number, device number, ID card number, etc.) Obtain the corresponding original data from the data storage layer, process the original data through the feature processing system to obtain th...
Embodiment 3
[0026] As a kind of application scheme of embodiment 1, such as figure 1 As shown, it includes the following steps: 1. Build a set of risk control system based on Internet big data, including data collection section, data storage terminal, risk control rule system, feature calculation system, model estimation system 2. Build a set of offline Feature model processing system, including data warehouse, offline feature mining system, model offline training system 3, online feature calculation system and offline feature mining system, using the same feature calculation lib 4, all online data are stored in the offline data warehouse as snapshots 5. To implement new feature mining, you only need to update the feature calculation lib, and then perform data mining and model making offline. 6. The new model produced and the updated new feature calculation lib can be launched at the same time, and the new features can be applied to the line.
[0027] Compared with the prior art, the pre...
PUM
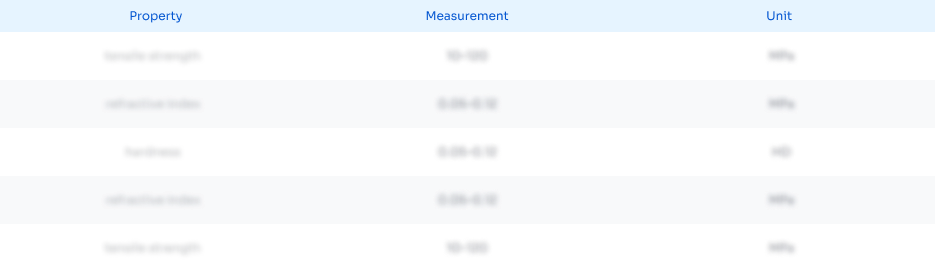
Abstract
Description
Claims
Application Information
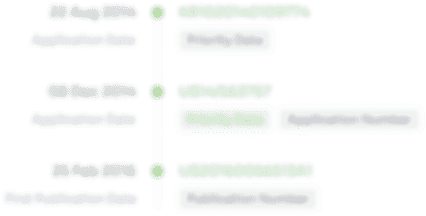
- R&D Engineer
- R&D Manager
- IP Professional
- Industry Leading Data Capabilities
- Powerful AI technology
- Patent DNA Extraction
Browse by: Latest US Patents, China's latest patents, Technical Efficacy Thesaurus, Application Domain, Technology Topic, Popular Technical Reports.
© 2024 PatSnap. All rights reserved.Legal|Privacy policy|Modern Slavery Act Transparency Statement|Sitemap|About US| Contact US: help@patsnap.com