Bearing fault diagnosis and prediction method based on extended Kalman filtering algorithm
An extended Kalman filter algorithm technology, which is applied in the field of bearing fault diagnosis and prediction based on the extended Kalman filter algorithm, can solve the problems of short time consumption, long time consumption, and low prediction accuracy
- Summary
- Abstract
- Description
- Claims
- Application Information
AI Technical Summary
Problems solved by technology
Method used
Image
Examples
Embodiment Construction
[0057] The present invention will be further described below in conjunction with the accompanying drawings.
[0058] refer to Figure 1 to Figure 9 , a bearing fault diagnosis and prediction method based on an extended Kalman filter algorithm, the method includes the following steps:
[0059] S1. Collect the vibration signal of the whole life cycle of the bearing;
[0060] S2. Use the vibration signal when the bearing is healthy to construct an AR model, use the AR model to filter the collected vibration signal, and highlight the information related to the vibration signal and the fault, so as to facilitate the construction of the subsequent health index, fault diagnosis and prediction;
[0061] S3. Use wavelet packet transform to analyze the residual signal after AR model filtering, and construct the energy feature corresponding to the wavelet packet coefficient for Mahalanobis distance calculation;
[0062] S4. Carry out the calculation of the Mahalanobis distance, and con...
PUM
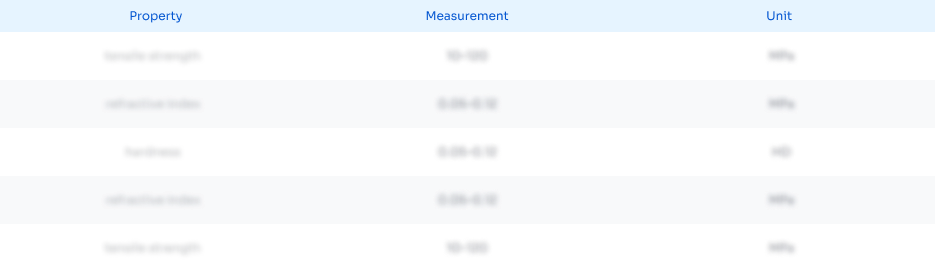
Abstract
Description
Claims
Application Information
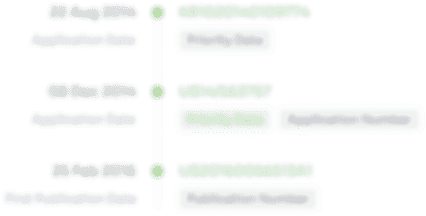
- R&D Engineer
- R&D Manager
- IP Professional
- Industry Leading Data Capabilities
- Powerful AI technology
- Patent DNA Extraction
Browse by: Latest US Patents, China's latest patents, Technical Efficacy Thesaurus, Application Domain, Technology Topic, Popular Technical Reports.
© 2024 PatSnap. All rights reserved.Legal|Privacy policy|Modern Slavery Act Transparency Statement|Sitemap|About US| Contact US: help@patsnap.com