Short-term load forecasting method based on artificial neural network improved training strategy
An artificial neural network and short-term load forecasting technology, applied in forecasting, data processing applications, instruments, etc., can solve the problems of reduced forecasting accuracy, long load forecasting time, poor robustness of forecasting methods, etc., to improve forecasting accuracy and speed, The effect of simple operation of load forecasting and flexible network selection
- Summary
- Abstract
- Description
- Claims
- Application Information
AI Technical Summary
Problems solved by technology
Method used
Image
Examples
Embodiment Construction
[0038] The present invention is described in detail below in conjunction with accompanying drawing:
[0039] Such as figure 1 As shown, the specific training strategy of the improved Elman artificial neural network is as follows: the input training sample takes the load value of N time before / after M days before the short-term load to be predicted as the input value, and the load at this time of the M days before the time to be predicted is the value of each group The entered central value is fed into the predictive model for prediction. The output of the forecasting model is the forecasted load value at N moments before / after the day to be forecasted, and the central value of this group of data is the load at the time to be forecasted on the day to be forecasted.
[0040] Such as figure 2 As shown, the model training and prediction process steps of the present invention are as follows:
[0041] Step (1): Establish the Elman artificial neural network, set the number of neu...
PUM
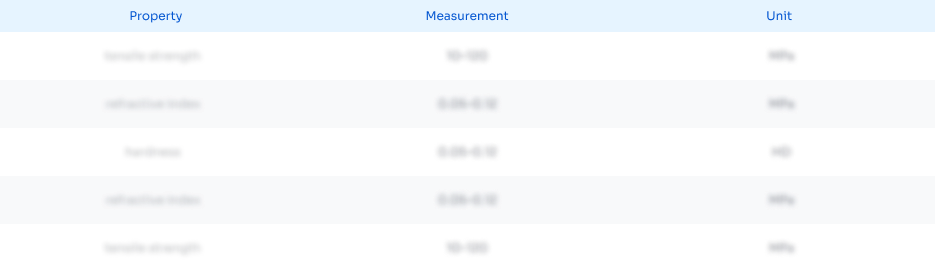
Abstract
Description
Claims
Application Information
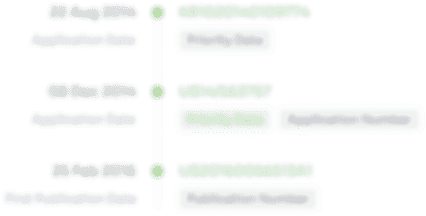
- R&D Engineer
- R&D Manager
- IP Professional
- Industry Leading Data Capabilities
- Powerful AI technology
- Patent DNA Extraction
Browse by: Latest US Patents, China's latest patents, Technical Efficacy Thesaurus, Application Domain, Technology Topic, Popular Technical Reports.
© 2024 PatSnap. All rights reserved.Legal|Privacy policy|Modern Slavery Act Transparency Statement|Sitemap|About US| Contact US: help@patsnap.com