PCM (possibilistic C-means) clustering algorithm based online automotive suspension performance monitoring method
A car suspension and mean value clustering technology, which is applied in computing, special data processing applications, instruments, etc., can solve problems such as large amount of computing and cannot realize online performance monitoring, and achieve high realizability, low online computing amount, and real strong effect
- Summary
- Abstract
- Description
- Claims
- Application Information
AI Technical Summary
Problems solved by technology
Method used
Image
Examples
specific Embodiment approach 1
[0013] Specific embodiment one: the on-line automobile suspension performance monitoring method based on possibility C-means clustering algorithm of the present embodiment, it realizes according to the following steps:
[0014] Step 1: Collect N accelerometer samples to obtain normal data when the vehicle suspension is in a healthy state; wherein each accelerometer sample is a four-dimensional vector, one accelerometer sample contains four accelerometer sample values, and each accelerometer sample The value is called an element of the accelerometer sample, each element represents an accelerometer value;
[0015] Step 2: Use the FCM algorithm to calculate the cluster centers of N normal data, use the calculated cluster centers as the initial value of PCM, and calculate the cluster center υ of PCM k and weight η i ; (The first two steps are the offline learning process, followed by the online monitoring process)
[0016] Step 3: During the operation of the car suspension, the ...
specific Embodiment approach 2
[0019] Specific implementation mode two: the difference between this implementation mode and specific implementation mode one is: FCM calculation is used in the second step
[0020] Calculate the cluster centers of N normal data using the method:
[0021] FCM is not sensitive to initial conditions, X={x i |i=1,2,…,N} is a data set composed of N accelerometer samples, one of which is represented by x i =[x i1 …x in ],x i is a sampling point, there are b data in a sampling point, x in is the bth data;
[0022] FCM completes the clustering of N samples by finding the solution that minimizes the objective function. The objective function is as follows,
[0023] J = Σ k = 1 c Σ i = 1 N ( μ k ...
specific Embodiment approach 3
[0029] Specific embodiment three: the difference between this embodiment and specific embodiment one or two is: said step two uses the calculated cluster center as the initial value of PCM, and calculates the cluster center v of PCM k and weight η i Specifically:
[0030] PCM is sensitive to initial conditions. The initial clustering results of FCM are used as the initial value of PCM. When PCM classifies accelerometer sample points, the membership degree of sample points to any clustering category is between 0 and 1. For all The sum of the membership degrees of categories is not 1, therefore, a data sample that does not belong to any known class has a small membership degree for each category;
[0031] PCM achieves clustering by finding the result with the smallest objective function. The objective function is as follows,
[0032] J = Σ i = 1 N ...
PUM
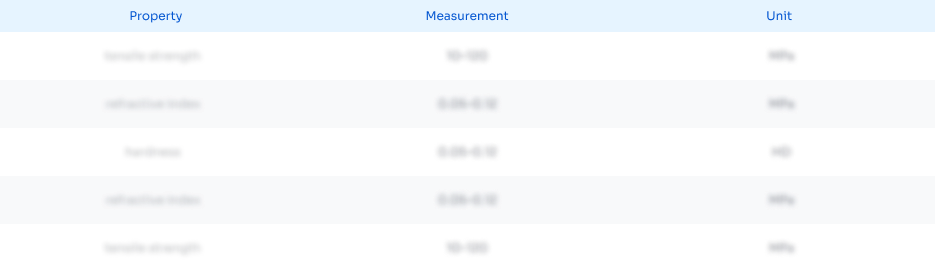
Abstract
Description
Claims
Application Information
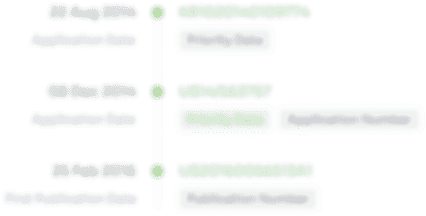
- R&D Engineer
- R&D Manager
- IP Professional
- Industry Leading Data Capabilities
- Powerful AI technology
- Patent DNA Extraction
Browse by: Latest US Patents, China's latest patents, Technical Efficacy Thesaurus, Application Domain, Technology Topic, Popular Technical Reports.
© 2024 PatSnap. All rights reserved.Legal|Privacy policy|Modern Slavery Act Transparency Statement|Sitemap|About US| Contact US: help@patsnap.com