Hyper-spectral image classification method based on non-local similarity and sparse coding
A non-local similarity, hyperspectral image technology, applied in the field of image processing, can solve problems such as the inability to obtain neighborhood information and the poor classification effect of homogeneous regions.
- Summary
- Abstract
- Description
- Claims
- Application Information
AI Technical Summary
Problems solved by technology
Method used
Image
Examples
Embodiment 1
[0065] like figure 1 As shown, the hyperspectral image classification method based on non-local similarity and sparse coding includes the following steps:
[0066] (1) Input hyperspectral image:
[0067] Input the hyperspectral image to be classified, which contains n pixels in total, set each pixel in the input hyperspectral image as a sample, and obtain the sample X of the hyperspectral image=[x 1 ,x 2 ,...,x p ,...,x n ]∈R d ,1≤p≤n, where d is the band number of the hyperspectral image, x p represents the p-th sample of a hyperspectral image, R d Represents a d-dimensional real number vector space;
[0068] (2) Non-local mean filtering:
[0069] The first step is to select a test sample x i , with x i As the center, set a 7×7 neighborhood window;
[0070] The second step is to set a filter window with a size of 3×3, and perform mean filtering on the samples in the neighborhood window;
[0071] The third step is to calculate the test sample x according to the fol...
Embodiment 2
[0110] In this embodiment, on the basis of Embodiment 1, the effects of the present invention are further described in combination with simulation diagrams.
[0111] 1. Simulation experiment conditions:
[0112] The hardware test platform of this experiment is: the processor is Intel Core2 CPU, the main frequency is 2.33GHz, the memory is 2GB, and the software platform is: Windows XP operating system and Matlab R2012a. The input image of the present invention is a hyperspectral image Indian Pines, the image size is 145×145×220, the image contains 220 bands and 16 types of ground objects, and the image format is TIF.
[0113] 2. Simulation content:
[0114] The three prior art comparative classification methods used in the present invention are respectively as follows:
[0115] Hyperspectral image classification proposed by Melgani et al. method, referred to as the support vector machine SVM classification method;
[0116] The hyperspectral image classification method propo...
PUM
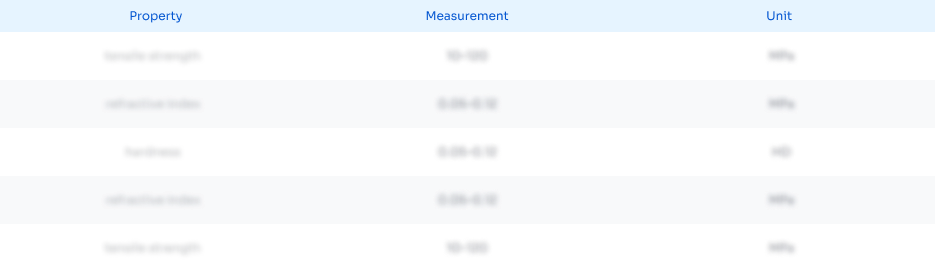
Abstract
Description
Claims
Application Information
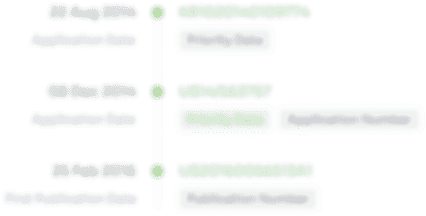
- R&D Engineer
- R&D Manager
- IP Professional
- Industry Leading Data Capabilities
- Powerful AI technology
- Patent DNA Extraction
Browse by: Latest US Patents, China's latest patents, Technical Efficacy Thesaurus, Application Domain, Technology Topic, Popular Technical Reports.
© 2024 PatSnap. All rights reserved.Legal|Privacy policy|Modern Slavery Act Transparency Statement|Sitemap|About US| Contact US: help@patsnap.com