Method for semantically annotating images on basis of hybrid generative and discriminative learning models
A learning model and semantic annotation technology, applied in character and pattern recognition, special data processing applications, instruments, etc., can solve problems such as inconvenient prior knowledge, achieve scalability, solve weak labeling problems, run efficiency and classification high precision effect
- Summary
- Abstract
- Description
- Claims
- Application Information
AI Technical Summary
Problems solved by technology
Method used
Image
Examples
Embodiment Construction
[0031] A hybrid generative and discriminative model for automatic image annotation. In the generative learning stage, continuous PLSA is used to generate generative modeling of images, which can make full use of the prior knowledge of the training set, and can obtain the corresponding model parameters and the topic distribution of each image; then use this topic distribution as each image The intermediate representation vector of the image, then the problem of automatic image labeling is transformed into a classification problem based on multi-label learning to obtain higher labeling accuracy than the generative model. In the discriminative learning stage, the method of constructing a cluster classifier chain is used to conduct discriminative learning on the intermediate representation vector of the image. When the classifier chain is established, the context information between the labeled keywords is also integrated, so that the image can be classified At the same time, it a...
PUM
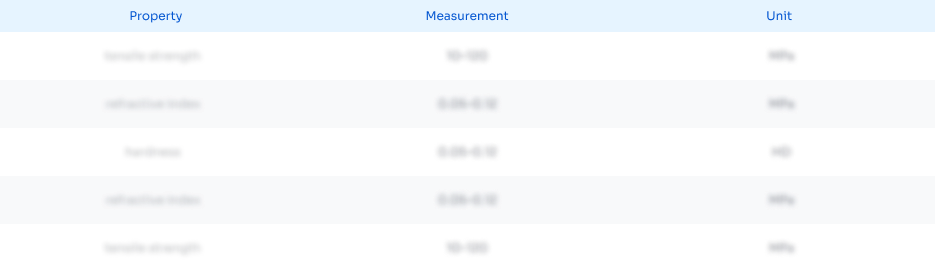
Abstract
Description
Claims
Application Information
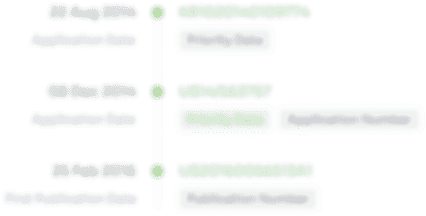
- R&D Engineer
- R&D Manager
- IP Professional
- Industry Leading Data Capabilities
- Powerful AI technology
- Patent DNA Extraction
Browse by: Latest US Patents, China's latest patents, Technical Efficacy Thesaurus, Application Domain, Technology Topic.
© 2024 PatSnap. All rights reserved.Legal|Privacy policy|Modern Slavery Act Transparency Statement|Sitemap