Image sorting method and system for active learning
A technology of active learning and classification method, applied in character and pattern recognition, instruments, computer parts and other directions, can solve the problems of large sampling workload, time-consuming and laborious, achieve high uncertainty and representativeness, improve processing efficiency, The effect of reducing sample processing time and effort
- Summary
- Abstract
- Description
- Claims
- Application Information
AI Technical Summary
Problems solved by technology
Method used
Image
Examples
Embodiment 1
[0056] The embodiment of the present invention discloses an active learning image classification method, please refer to figure 1 , the method includes the following steps:
[0057] S1: Analyze and process the original unlabeled image sample set to obtain the most uncertain image sample set including at least one image sample, and each image sample in the most uncertain image sample set corresponds to a characterizing The first parameter of the degree of uncertainty of the Z image category, the parameter value of the first parameter satisfies the preset condition that characterizes the high uncertainty of the image sample, wherein the Z is a natural number greater than 1.
[0058] In this embodiment, the uncertainty and representativeness of the image samples are comprehensively considered, and the image samples with higher uncertainty and higher representativeness are taken as image samples with higher information content, that is, for the preset Z image categories, the most ...
Embodiment 2
[0110] Each of the image samples to be labeled (that is, each image sample in the representative image sample set) screened in the first embodiment above has both high uncertainty and high representativeness. In the second embodiment, based on the samples to be labeled After having the above two advantages, continue to optimize the samples to be labeled, and use the expected error rate reduction strategy to select the most informative samples from the samples to be labeled.
[0111] The core idea of the expected error rate reduction strategy is: for each image sample in all candidate image samples, add the image sample to the labeled image sample set (that is, the labeled image sample set that trains the current image classifier) , and use the added labeled image sample set to update the current image classifier to obtain a new classifier; after that, use the new classifier to classify the remaining candidate image samples in the candidate image samples, and calculate the new...
Embodiment 3
[0127] Embodiment 3 of the present invention discloses an active learning image classification system, which corresponds to the active learning image classification methods in Embodiment 1 and Embodiment 2.
[0128] First, corresponding to the flow of the active learning image classification method in the first embodiment, the second embodiment discloses a structure of the active learning image classification system, please refer to Figure 6 , the system includes a first sampling module 100 , a second sampling module 200 , a labeling module 300 , a training module 400 and a classification module 500 .
[0129] The first sampling module 100 is configured to analyze and process the original unlabeled image sample set to obtain the most uncertain image sample set including at least one image sample, and each image sample in the most uncertain image sample set corresponds to one A first parameter that characterizes its degree of uncertainty relative to the preset Z image categori...
PUM
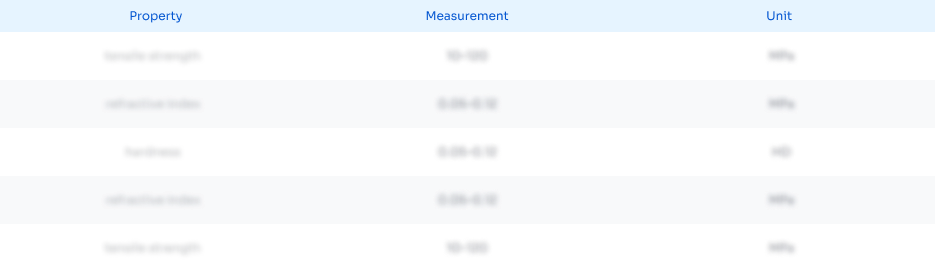
Abstract
Description
Claims
Application Information
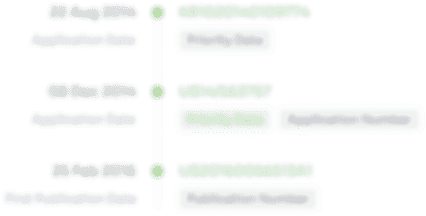
- R&D Engineer
- R&D Manager
- IP Professional
- Industry Leading Data Capabilities
- Powerful AI technology
- Patent DNA Extraction
Browse by: Latest US Patents, China's latest patents, Technical Efficacy Thesaurus, Application Domain, Technology Topic.
© 2024 PatSnap. All rights reserved.Legal|Privacy policy|Modern Slavery Act Transparency Statement|Sitemap