EMD (Empirical Mode Decomposition) endpoint effect suppression method based on HMM (Hidden Markov Model) correction and neural network extension
A neural network and endpoint effect technology, applied in the field of signal processing, to achieve the effect of solving distortion and suppressing endpoint effect
- Summary
- Abstract
- Description
- Claims
- Application Information
AI Technical Summary
Problems solved by technology
Method used
Image
Examples
Embodiment Construction
[0045] The specific implementation manners of the present invention will be further described in detail below in conjunction with the accompanying drawings and embodiments. The following examples are used to illustrate the present invention, but are not intended to limit the scope of the present invention.
[0046] An embodiment of the EMD endpoint effect suppression method based on HMM correction and neural network extension of the present invention, its flow chart is as follows figure 1 As shown, the specific content includes the following steps:
[0047] Step s101, using sensors to acquire vibration signals;
[0048] Step s102, using the neural network continuation algorithm to estimate part of the known data inside the signal endpoint, calculate the estimation error, and predict the data outside the endpoint, including the following steps:
[0049] (1) Use neural network prediction technology to predict data other than endpoints to obtain a prediction sequence ...
PUM
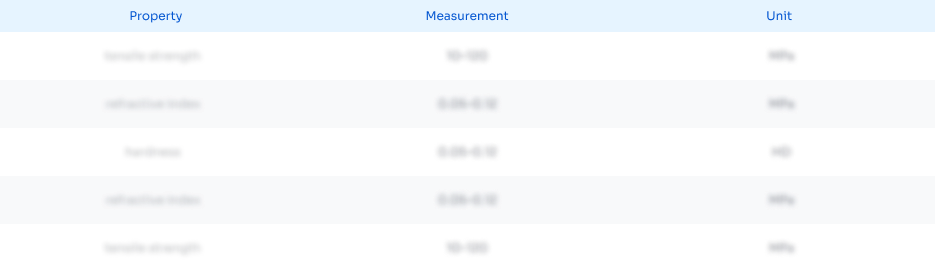
Abstract
Description
Claims
Application Information
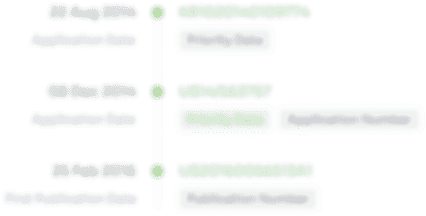
- R&D Engineer
- R&D Manager
- IP Professional
- Industry Leading Data Capabilities
- Powerful AI technology
- Patent DNA Extraction
Browse by: Latest US Patents, China's latest patents, Technical Efficacy Thesaurus, Application Domain, Technology Topic, Popular Technical Reports.
© 2024 PatSnap. All rights reserved.Legal|Privacy policy|Modern Slavery Act Transparency Statement|Sitemap|About US| Contact US: help@patsnap.com