Missing label multi-label classification method based on example-level and label-level association
A classification method and tag-level technology, applied in the field of multi-label classification, can solve problems such as difficulty in determining the σ value of the Gaussian function, inaccurate similarity, and negative impact on algorithm results, etc., to achieve improved classification effect, accurate tag association, and good effect Effect
- Summary
- Abstract
- Description
- Claims
- Application Information
AI Technical Summary
Problems solved by technology
Method used
Image
Examples
Embodiment Construction
[0025] The technical solutions in the embodiments of the present invention will be described clearly and in detail below with reference to the drawings in the embodiments of the present invention. The described embodiments are only some of the embodiments of the invention.
[0026] The technical scheme that the present invention solves the problems of the technologies described above is:
[0027] Specific steps:
[0028] Step S1: Input the feature matrix of the training sample Consists of n examples, each represented as a d-dimensional feature vector. Assume that each sample is linearly related to its neighbors, that is to say, each sample can be reorganized by a linear combination of its neighbors, namely represents the sample x i The k nearest neighbor samples of . Then, the weight matrix W can be obtained by solving the following quadratic programming problem:
[0029]
[0030] The weight matrix W obtained by the above formula is not symmetrical. In order to obta...
PUM
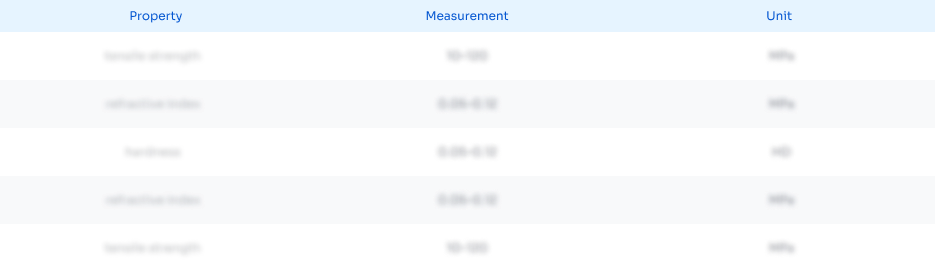
Abstract
Description
Claims
Application Information
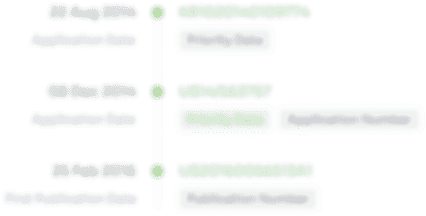
- R&D Engineer
- R&D Manager
- IP Professional
- Industry Leading Data Capabilities
- Powerful AI technology
- Patent DNA Extraction
Browse by: Latest US Patents, China's latest patents, Technical Efficacy Thesaurus, Application Domain, Technology Topic, Popular Technical Reports.
© 2024 PatSnap. All rights reserved.Legal|Privacy policy|Modern Slavery Act Transparency Statement|Sitemap|About US| Contact US: help@patsnap.com