Semi-supervised classification method for hyperspectral remote sensing images based on local manifold learning composition
A technology of hyperspectral remote sensing and classification methods, which is applied to computer parts, instruments, character and pattern recognition, etc., and can solve problems such as poor reconstruction effect, insufficient use of local structural information of data points, and large amount of calculation
- Summary
- Abstract
- Description
- Claims
- Application Information
AI Technical Summary
Problems solved by technology
Method used
Image
Examples
Embodiment 1
[0054] Use the GFHF_LLE method.
[0055] combine figure 1 , the technical scheme that the present invention adopts for solving its technical problem is: provide a kind of hyperspectral remote sensing image semi-supervised classification method based on local manifold learning composition, specifically comprise the following steps:
[0056] (1) Select training data set X and test data set X t , the training dataset X includes a labeled dataset X m and the unlabeled dataset X u :
[0057] where X m is the set of m labeled data points in the hyperspectral remote sensing image, X m The tag information of Y m Represented by a matrix with a size of C×m, C is the number of categories of object types, Y m The value Y of the element in row i and column j in ij Used to indicate the j-th labeled data point, if the j-th labeled data point belongs to the i-th class, then Y ij = 1, otherwise Y ij = 0;
[0058] x u is a set of randomly selected part of the unlabeled data points i...
Embodiment 2
[0080] The GFHF_LTSA method is adopted, which specifically includes the following steps:
[0081] (1) Select training data set X and test data set X t , the training dataset X includes a labeled dataset X m and the unlabeled dataset X u :
[0082] where X m is the set of m labeled data points in the hyperspectral remote sensing image, X m The tag information of Y m Represented by a matrix with a size of C×m, C is the number of categories of object types, Y m The value Y of the element in row i and column j in ij Used to indicate the j-th labeled data point, if the j-th labeled data point belongs to the i-th class, then Y ij = 1, otherwise Y ij = 0;
[0083] x u is a set of randomly selected part of the unlabeled data points in the hyperspectral remote sensing image, the number is u, u>>m;
[0084] The test data set X t X in the hyperspectral remote sensing image u A collection of unlabeled data points other than ;
[0085] (2) Calculate the unlabeled data set X ...
PUM
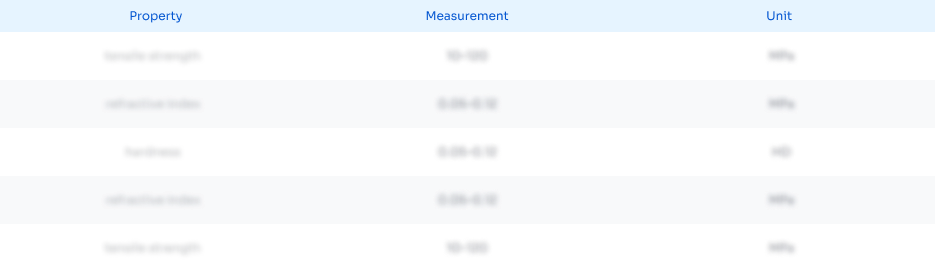
Abstract
Description
Claims
Application Information
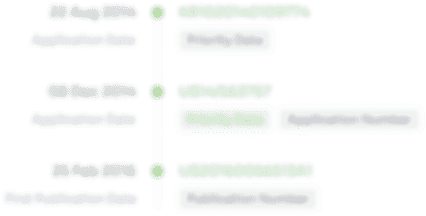
- R&D Engineer
- R&D Manager
- IP Professional
- Industry Leading Data Capabilities
- Powerful AI technology
- Patent DNA Extraction
Browse by: Latest US Patents, China's latest patents, Technical Efficacy Thesaurus, Application Domain, Technology Topic, Popular Technical Reports.
© 2024 PatSnap. All rights reserved.Legal|Privacy policy|Modern Slavery Act Transparency Statement|Sitemap|About US| Contact US: help@patsnap.com