Prediction method of algal blooms in lakes and reservoirs based on multivariate non-stationary time series analysis, neural network and support vector machine compensation
A technology of time series analysis and support vector machine, which is applied in special data processing applications, instruments, electrical digital data processing, etc., and can solve problems such as low accuracy of algae bloom prediction, prediction, and difficult small sample data
- Summary
- Abstract
- Description
- Claims
- Application Information
AI Technical Summary
Problems solved by technology
Method used
Image
Examples
Embodiment 1
[0113] Step 1. Multivariate non-stationary time series modeling of characteristic factors;
[0114] Nine water bloom characteristic factors were monitored from June 2009 to June 2012 in Taihu Lake, Jiangsu Province, see Table 1 for details.
[0115] Table 1 Monitoring list of characteristic factors of water bloom
[0116] name
[0117] Among them, chlorophyll is the characterization factor of water bloom, and the other 8 characteristic factors are the influencing factors of water bloom. The monitoring equipment recorded a total of 1050 days of water bloom characteristic factor data, and the 901-day monitoring data after the original time series of 9 characteristic factors were zero-meanized was used for multivariate non-stationary time-series modeling, and the characteristic factors from 901 days to 1050 days For multivariate non-stationary time-series prediction of chlorophyll, see image 3 and Figure 4 .
[0118] In order to compare the error prediction result...
PUM
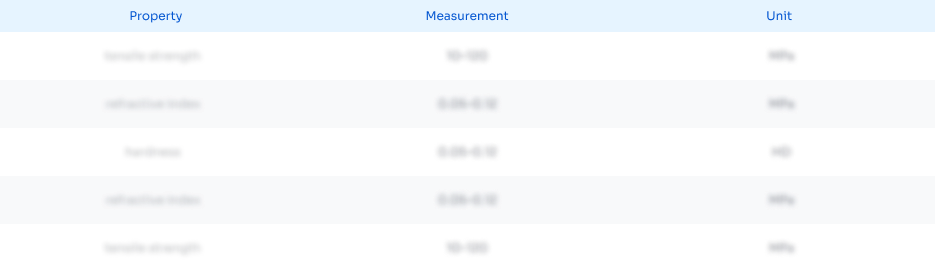
Abstract
Description
Claims
Application Information
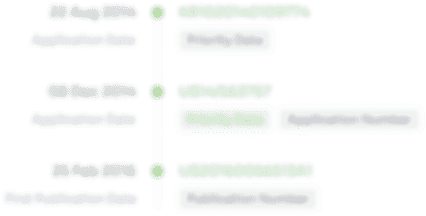
- R&D Engineer
- R&D Manager
- IP Professional
- Industry Leading Data Capabilities
- Powerful AI technology
- Patent DNA Extraction
Browse by: Latest US Patents, China's latest patents, Technical Efficacy Thesaurus, Application Domain, Technology Topic, Popular Technical Reports.
© 2024 PatSnap. All rights reserved.Legal|Privacy policy|Modern Slavery Act Transparency Statement|Sitemap|About US| Contact US: help@patsnap.com