Human face recognition method based on multiple-feature space sparse classifiers
A sparse classifier and feature space technology, applied in the field of face recognition, can solve problems such as poor generalization ability and inability to make full use of features
- Summary
- Abstract
- Description
- Claims
- Application Information
AI Technical Summary
Problems solved by technology
Method used
Image
Examples
Embodiment Construction
[0034] In order to make the purpose of the present invention, implementation scheme and advantage clearer, the specific implementation of the present invention is described in further detail below, and the concrete process of the present invention is as follows figure 1 shown.
[0035] (1) The original training sample {X 1 …X N} projected to the Eigenface feature space to form a sub-dictionary O E , the sample vector expression after projection in the feature space is Y K =W PCA T x K ,X K is a training sample vector, W PCA It is the matrix composed of the bases of the Eigenface feature space, and the set {Y 1 ...Y K ...Y N} is the sub-dictionary O E .
[0036] (2) The original training sample {X 1 …X N} projected to the Laplacianface feature space to form a sub-dictionary O E , the sample vector expression after projection in the feature space is Y K =W T x K ,W=W PCA W LPP , W PCA Indicates that principal vector analysis is first performed on the origina...
PUM
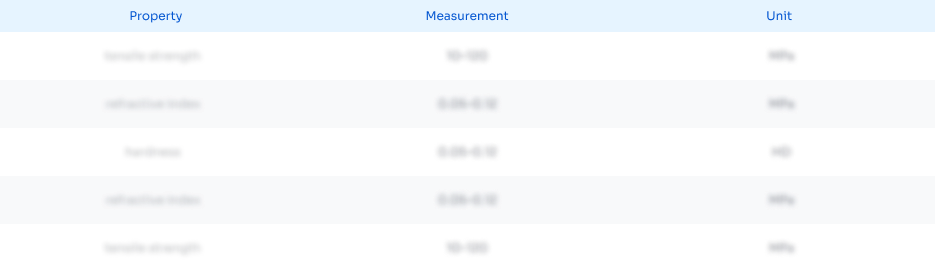
Abstract
Description
Claims
Application Information
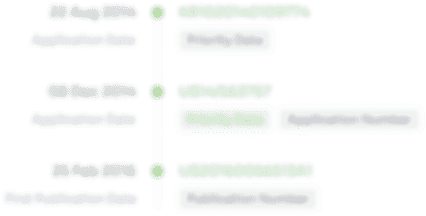
- R&D Engineer
- R&D Manager
- IP Professional
- Industry Leading Data Capabilities
- Powerful AI technology
- Patent DNA Extraction
Browse by: Latest US Patents, China's latest patents, Technical Efficacy Thesaurus, Application Domain, Technology Topic.
© 2024 PatSnap. All rights reserved.Legal|Privacy policy|Modern Slavery Act Transparency Statement|Sitemap