Super-resolution image reconstruction method based on non-local dictionary learning and biregular terms
A super-resolution reconstruction and non-local dictionary technology, applied in the field of image processing, can solve problems such as image artifacts, ignoring prior knowledge of ultra-low resolution images, distortion, etc., to improve accuracy, maintain edge and texture details , the effect of improving quality
- Summary
- Abstract
- Description
- Claims
- Application Information
AI Technical Summary
Problems solved by technology
Method used
Image
Examples
Embodiment Construction
[0023] Refer to attached figure 1 , concrete steps of the present invention include:
[0024] Step 1. To the initial high-resolution image Carry out adaptive clustering dictionary training to get R cluster centers C center ={C i ,i=1,2,...,R}, the initial expected dictionary set D 0 and the initial set of residual dictionaries d 0 .
[0025] 1a) Extract the initial high-resolution image The high-frequency features of , get the high-frequency feature map G;
[0026] 1b) respectively in the initial high-resolution image Take a 7×7 block on the high-frequency feature image G, and the initial high-resolution image All the image blocks obtained above are arranged sequentially in the form of column vectors to form a set of image blocks Arrange all the feature blocks acquired on the high-frequency feature image G in the form of column vectors to form a set of feature blocks
[0027] 1c) Use K-means clustering method to set feature blocks Perform clustering to get R...
PUM
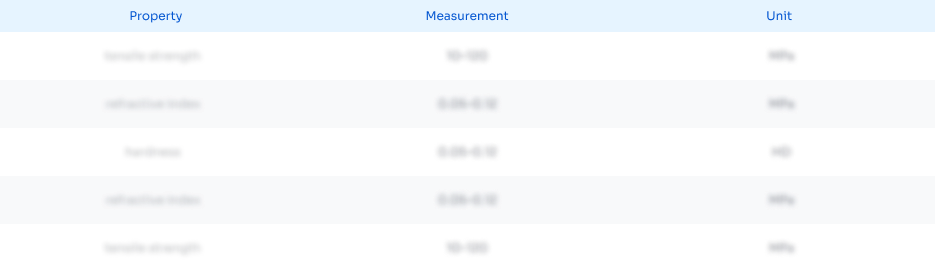
Abstract
Description
Claims
Application Information
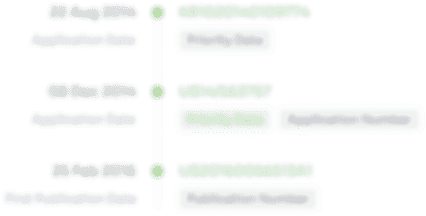
- Generate Ideas
- Intellectual Property
- Life Sciences
- Materials
- Tech Scout
- Unparalleled Data Quality
- Higher Quality Content
- 60% Fewer Hallucinations
Browse by: Latest US Patents, China's latest patents, Technical Efficacy Thesaurus, Application Domain, Technology Topic, Popular Technical Reports.
© 2025 PatSnap. All rights reserved.Legal|Privacy policy|Modern Slavery Act Transparency Statement|Sitemap|About US| Contact US: help@patsnap.com