A Domain Adaptive Image Classification Method Based on Hybrid Pooling
A classification method, a hybrid pool technology, applied in the fields of instruments, computing, character and pattern recognition, etc., can solve the problems of deepening the degree of network overfitting, inability to recognize and classify objects, and insufficient abstraction of extracted features, and achieve image features. Abstract and complete, solve generalization problems, improve the effect of generalization
- Summary
- Abstract
- Description
- Claims
- Application Information
AI Technical Summary
Problems solved by technology
Method used
Image
Examples
Embodiment Construction
[0029] The present invention will be further described below in conjunction with the examples, but not as a limitation of the present invention.
[0030] The field adaptive image classification method based on hybrid pooling of the present invention comprises the following steps:
[0031] Send the samples in the target domain test set to the trained image classification prediction model, please combine figure 1 The image classification prediction model shown includes the first convolutional layer C1 and the second convolutional layer C2 connected in sequence, the second convolutional layer is connected to the maximum pooling layer P1, and the maximum pooling layer P1 is cascaded with a layer of average pooling layer P2 , the average pooling layer P2 is connected to the fully connected layer fc with a softmax activation function.
[0032] The samples in the test set of the target domain first enter the first convolutional layer C1 to extract the underlying features in the imag...
PUM
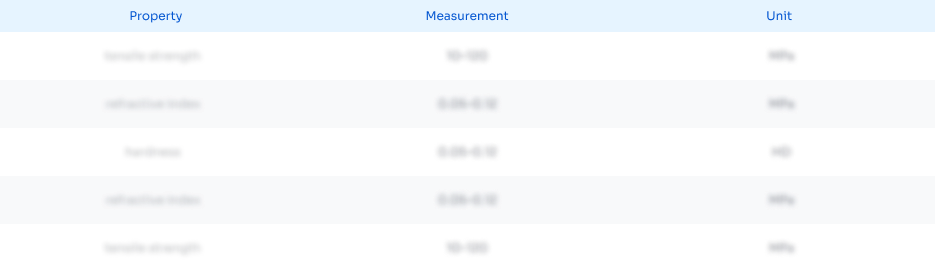
Abstract
Description
Claims
Application Information
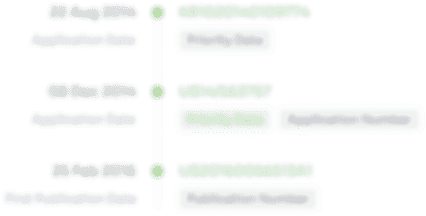
- R&D
- Intellectual Property
- Life Sciences
- Materials
- Tech Scout
- Unparalleled Data Quality
- Higher Quality Content
- 60% Fewer Hallucinations
Browse by: Latest US Patents, China's latest patents, Technical Efficacy Thesaurus, Application Domain, Technology Topic, Popular Technical Reports.
© 2025 PatSnap. All rights reserved.Legal|Privacy policy|Modern Slavery Act Transparency Statement|Sitemap|About US| Contact US: help@patsnap.com