
Artificial Intelligence (AI) is no longer just a tech industry buzzword – it has become a driving force in scientific discovery. From biology labs to climate science centers, researchers are increasingly relying on AI tools and models to uncover insights that were previously out of reach. In fact, many scientists now describe AI’s emergence as “a revolution for research,” with surveys confirming that university students and researchers use AI on a weekly or even daily basis. This revolution spans both the process of doing research – for example, using chatbots to summarize literature or suggest experiments – and the substance of research, where AI algorithms are making landmark discoveries in materials science, biology, climate modeling, and more. In this comprehensive overview, we’ll explore real-world examples of how AI is transforming scientific research, highlight the latest developments, and discuss the opportunities and challenges that come with this new era of AI-assisted science.
Start Accelerating Your Scientific Discovery with PatSnap Eureka AI Agent
Unlock the full potential of your R&D with PatSnap’s Eureka AI Agent platform—a powerful, intelligent assistant designed to supercharge research in materials science, biology, and pharmaceuticals.
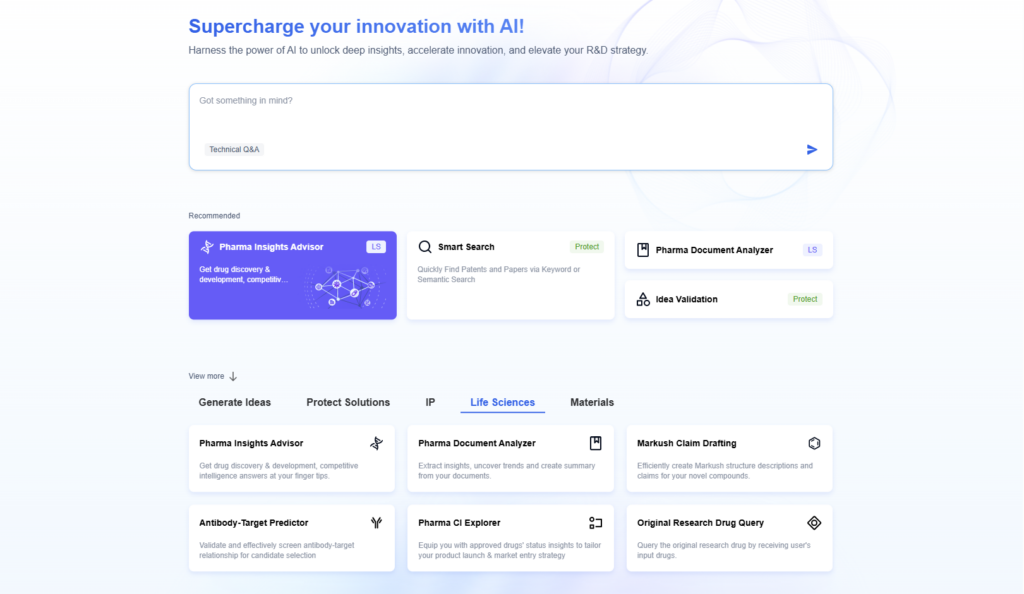
The AI Revolution in Scientific Discovery
AI continues to reshape scientific research across nearly every field. Machine learning algorithms find patterns in massive datasets with remarkable speed. They optimize complex simulations and even help generate new scientific hypotheses. These strengths complement human intuition and creativity, creating a powerful partnership. Researchers now describe this as a growing synergy between AI and science.
By framing scientific problems as computational challenges, scientists unlock AI’s cross-disciplinary power. Biologists use AI to label medical images and decode genomic data quickly. Chemists rely on it to analyze molecular structures and accelerate drug discovery. Physicists and engineers apply AI to refine simulations and test new materials. Cognitive scientists use language models to study how humans process language. In turn, AI design benefits from biology and neuroscience, forming a feedback loop of innovation.
At places like MIT, researchers train AI to detect data anomalies, predict group behavior, and design advanced robots. These tools help scientists move faster and ask deeper questions. This cycle of collaboration between AI and science promises to drive major discoveries for years to come.
Crucially, AI’s ability to learn from vast amounts of data enables it to tackle problems once considered intractable. In field after field, AI systems are uncovering patterns or solutions that humans alone might miss. Below, we delve into specific domains to see this impact in action.
Transforming Drug Discovery and Development with AI
Artificial intelligence is dramatically accelerating drug discovery by sifting through vast chemical spaces and biological data to find promising therapeutics much faster than traditional methods. One landmark example is the discovery of new antibiotics using machine learning. In 2020, an MIT team trained a deep-learning model on molecular structures and their antibacterial activity, then unleashed it on large chemical libraries. The AI identified a novel compound (later named halicin) that proved effective against some of the most dangerous drug-resistant bacteria. Halicin works via a unique mechanism unlike existing antibiotics, demonstrating how AI can uncover entirely new classes of drugs that humans might overlook. This was hailed as a milestone, being “a first” in antibiotic discovery via AI, and it underscored AI’s potential to tackle urgent health threats by rapidly proposing solutions from big data.
Accelerating Candidate Design and Clinical Trials
AI is dramatically speeding up the drug discovery process. In early 2020, the first AI-designed drug entered Phase I human trials. This molecule, created to treat obsessive-compulsive disorder, was developed by Exscientia and Sumitomo Dainippon Pharma. It reached human testing in under 12 months—a process that usually takes years.
Since then, AI-designed molecules from companies like Insilico Medicine and Exscientia have moved into clinical and preclinical stages. These early wins prove that AI can discover new chemistry and design better candidates faster. It cuts down the time spent on trial-and-error in traditional R&D.
A recent analysis reported around 160 drug programs now use AI-driven design. About 15 of these are already in clinical development. That’s roughly half the early-stage pipeline of major pharmaceutical firms.
This momentum marks a major shift. More future medicines will likely come from algorithms trained on biology and chemistry. These tools suggest drug candidates with speed and accuracy beyond human capacity.
Advancing Genomics, Bioinformatics, and Precision Medicine
Modern biology generates massive datasets, from genomes to clinical records, that overwhelm human analysis. AI helps turn this complexity into insight.
In genomics, machine learning can scan thousands of genomes to find disease-linked genes. It also predicts how genetic changes affect protein function. Deep learning models forecast gene expression and map regulatory networks with high accuracy.
These tools help researchers explore diseases at a molecular level. In precision medicine, AI combines genomic data, medical history, and lifestyle factors. It then recommends personalized treatments or flags candidates for drug trials.
AI has already delivered real results. Some algorithms can detect cancer subtypes using gene expression patterns. Others predict which mutations will respond to specific therapies.
These advances lead to more focused research and better outcomes. AI empowers scientists to decode human biology with unmatched speed and precision.
AlphaFold: A Breakthrough in Protein Structure Prediction
AlphaFold, developed by DeepMind, marked a breakthrough in bioinformatics by solving a decades-old scientific challenge. Its deep learning system predicts 3D protein structures from amino acid sequences with high accuracy.
For 50 years, researchers struggled with this problem. In 2021, AlphaFold stunned the science world by delivering the most accurate protein models ever. By 2022, it released predictions for over 200 million proteins from various organisms.
This database is now transforming biology. Scientists can instantly access predicted structures for proteins tied to disease, agriculture, or bioengineering. This speeds up critical research steps.
Researchers can now identify drug targets in days by studying pathogen proteins. They can also examine how mutations may change protein shapes and functions.
AlphaFold acts as a force multiplier in labs worldwide. It gives scientists a huge base of protein knowledge to boost discoveries and generate new ideas faster.
PatSnap Eureka AI Agent in Life Sciences Research
AI’s impact on biology isn’t limited to discovering new molecules or decoding genomes – it’s also changing how researchers access and apply scientific knowledge. The PatSnap Eureka AI Agent is one platform that exemplifies this in practice, acting as an intelligent research assistant for life scientists and IP (intellectual property) professionals. Trained on vast technical data (including millions of patents, scientific publications, clinical trial data, and biomedical databases), Eureka’s life sciences modules can answer complex R&D questions in seconds, helping researchers navigate information that would otherwise take weeks to gather.
Biomedical Q&A for Drug Development
Eureka offers a powerful Pharma Insights Advisor for drug development and biotech research. Scientists can ask natural-language questions and get quick, accurate answers.
Questions may cover drug mechanisms, FDA regulations, or clinical trial results. The system delivers evidence-based responses in seconds. Dr. Andrew Miller, a clinical research scientist, uses Eureka to explore drug development, regulations, investments, and clinical data.
He says the tool gives accurate answers and outperforms others he has tried. Eureka combines a strong AI model with PatSnap’s verified biomedical database.
Dr. Miller usually finds what he needs in just minutes. That speed makes a big difference. Instead of reading dozens of papers, researchers get the facts instantly.
Eureka supports faster literature reviews, competitive analysis, and compliance checks. It simplifies complex tasks and saves valuable research time.
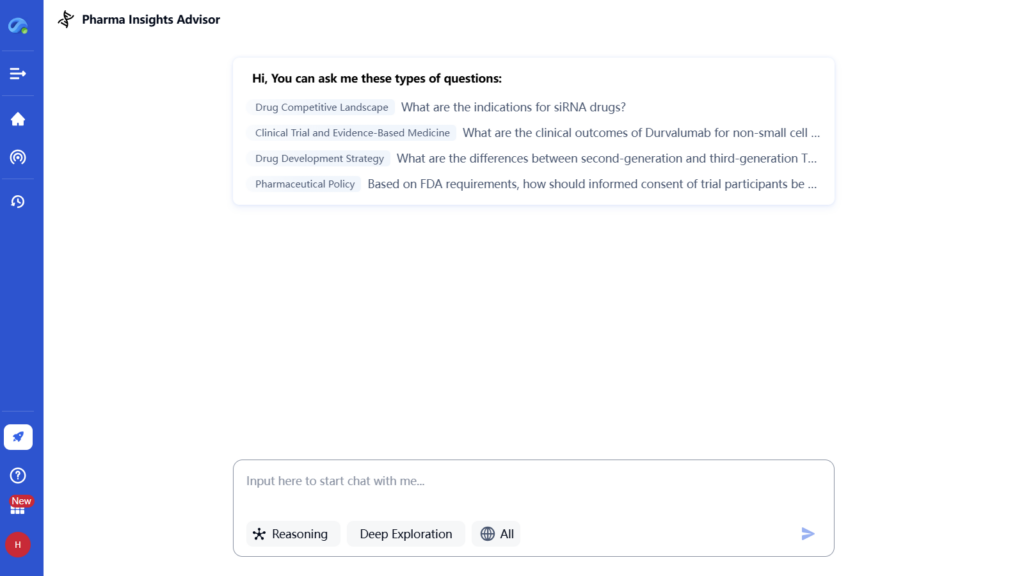
Mining Patents and Literature for Biopharma Innovation
Eureka provides a smart Pharma Insights Advisor for drug development and biotech research. Researchers ask natural questions and get fast, reliable answers.
Users can explore drug mechanisms, FDA rules, or clinical trial data within seconds. The AI delivers concise, evidence-backed responses instantly. Dr. Andrew Miller, a clinical scientist, relies on Eureka for daily research. He uses it to find data on drug development, regulations, and investments.
He reports high accuracy and better performance compared to other tools. Eureka blends advanced AI with PatSnap’s trusted biomedical content. Dr. Miller often gets the needed information in minutes. That speed transforms his workflow and saves valuable time.
Instead of scanning endless documents, scientists get clear answers fast. Eureka accelerates literature reviews, trend spotting, and regulatory tasks. This efficiency helps teams stay competitive and focus on innovation. Eureka makes research smarter, faster, and easier for biotech professionals.
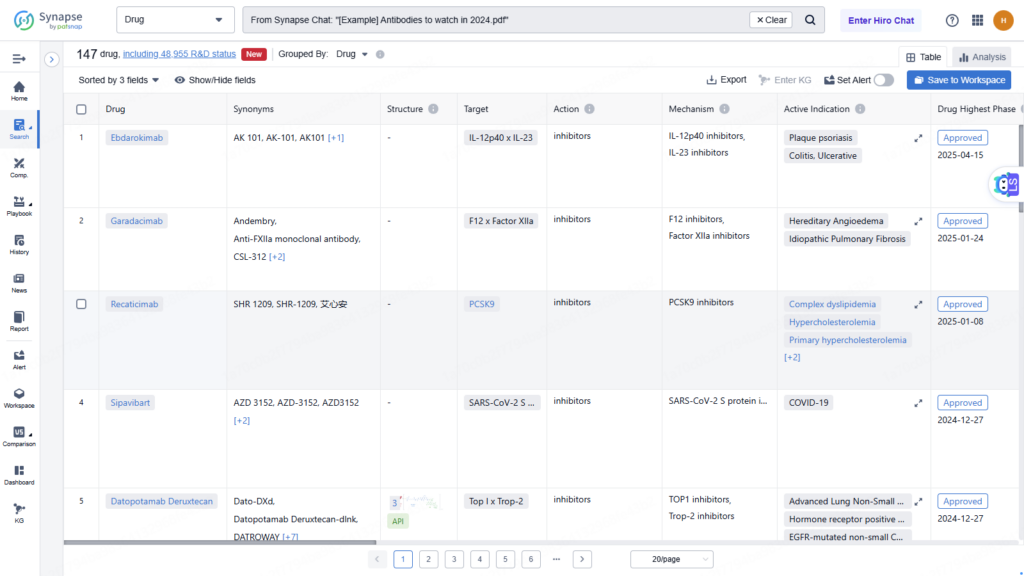
Automating Biological Sequence Analysis for IP
Another arena where the Eureka AI Agent contributes is biological sequence analysis for IP. In fields like genomics and biotechnology, verifying the novelty of gene or protein sequences is crucial for patenting and research. Traditionally, checking if a DNA or protein sequence has been patented or published is a labor-intensive search. Eureka’s specialized life science capabilities can rapidly compare sequences against patent databases and literature. PatSnap’s users report that they can “analyze hundreds of sequences and their prior art in a matter of minutes” – an enormous time savings. This speed is vital for companies racing to patent new biotech inventions or researchers ensuring their engineered gene is unique. By automating sequence searches, AI tools like Eureka free up scientists to focus on designing experiments and interpreting results, rather than wading through databases.
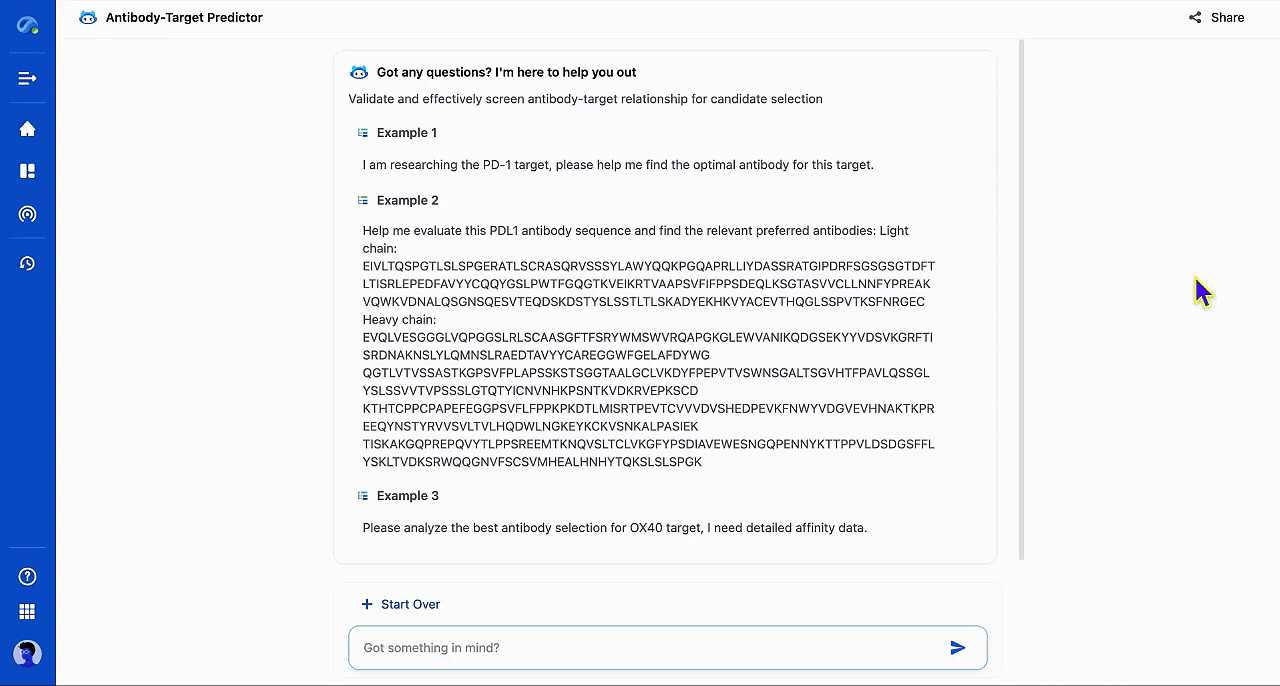
Driving Productivity and Innovation in Biotech
The PatSnap Eureka AI Agent acts as a powerful tool for life science research. It handles data gathering and speeds up early analysis. Researchers save time and make smarter decisions using Eureka. Productivity improves, and teams avoid wasted effort during early research. BioNTech, known for its COVID-19 vaccine, uses PatSnap tools to support breakthrough innovation. While projects stay confidential, the impact is clear. Eureka likely helps with mRNA patent searches and vaccine delivery insights. Leading biotech firms trust it to accelerate critical discoveries. PatSnap reports impressive results from its AI tools. Eureka boosts IP research productivity by up to 75% for many users. It also cuts R&D costs by about 25% on average. These savings help teams move faster and avoid expensive missteps. Eureka reduces AI-generated errors by 60% compared to generic models. Its answers come from trusted, well-curated biomedical data.
These improvements support quicker discovery, fewer dead ends, and better scientific decisions. Researchers reach insights faster and with more confidence. By using Eureka, teams transform how they work. Innovation becomes smarter, faster, and more accurate from start to finish.
AI-Driven Discovery of New Materials
Materials science is another field undergoing a revolution through artificial intelligence. Traditionally, discovering a novel material (whether a new alloy, polymer, or catalyst) could take years of trial-and-error experimentation and serendipity. AI is flipping this paradigm by enabling researchers to predict and screen material candidates virtually, pinpointing the most promising ones for lab testing in a fraction of the time.
Case Study: AI-Discovered Solid-State Electrolyte
A powerful illustration comes from a collaboration between Microsoft and the U.S. Pacific Northwest National Laboratory (PNNL). In 2024, they used advanced AI models to virtually screen over 32 million potential materials in search of a new solid-state electrolyte for better batteries. From that enormous search space, the AI identified about 500,000 stable candidates and ultimately pinpointed one highly promising material that did not exist in nature. Remarkably, PNNL scientists then synthesized and tested this AI-suggested material – and it worked. The new material demonstrated unique properties as a battery electrolyte, achieving similar performance to existing options while potentially using 70% less lithium (a critical benefit given lithium’s scarcity). By combining AI predictions with expert knowledge, the team compressed a discovery process that might have taken years into just weeks or days. This real-world success story shows AI acting as a catalyst for materials innovation: it can find that proverbial needle in the haystack (a material with just the right traits) at unprecedented speed, enabling faster development of technologies like safer batteries, more efficient solar panels, or stronger composites.
Discovering Record-Breaking Shape Memory Alloys
Beyond accelerating known search processes, AI can also uncover materials with record-breaking performance. For example, researchers at Texas A&M University applied a machine-learning framework to discover a new high-efficiency shape memory alloy – a material that can reversibly change shape with temperature. This alloy (a novel nickel-titanium composition) exhibited the highest operational efficiency ever recorded for its class. What’s notable is how AI changed the pace of innovation: historically, major advances in shape memory alloys occurred roughly once a decade due to the slow, iterative nature of experimentation. Using AI to guide the search dramatically shortened that cycle. The algorithm was able to explore the vast compositional design space and identify an optimal alloy that human researchers hadn’t found, all by recognizing hidden patterns in prior experimental data.
Balancing Multiple Properties with AI
This case underscores AI’s ability to optimize multiple objectives simultaneously – in materials design, often several properties (strength, weight, heat resistance, etc.) must be balanced, a task that becomes exponentially complex as options increase. AI algorithms excel at navigating such complexity, suggesting candidate materials that meet diverse criteria (for instance, an alloy that is both lightweight and ultra-strong and cost-effective). The outcome is not only faster discovery, but often better materials than what incremental tweaks would achieve.
Accelerating Materials R&D and Testing
Artificial intelligence is transforming daily operations in materials research, notably through the advent of “self-driving labs.” These automated laboratories leverage AI to instruct robots on specific experiments, analyze results instantly, and autonomously determine subsequent steps. This closed-loop process accelerates the discovery of optimal material formulations, significantly reducing the duration traditionally required for experimentation. Early implementations have successfully discovered advanced polymers and nanomaterials far quicker than conventional methods. By utilizing AI to predict experimental outcomes, these labs drastically reduce the number of physical trials necessary, saving substantial time and resources.
Streamlining Research with AI Assistants
Even without full automation, AI significantly enhances productivity by managing routine research tasks. Materials scientists frequently require comprehensive data on existing materials, including properties and market availability, which typically involves laborious literature and patent database searches. AI-driven tools compile this essential data rapidly, exemplified by Dow Chemical Company’s experience with PatSnap’s Eureka AI. During a beta test, Dow’s researchers reported a dramatic reduction in information-gathering time, from weeks to mere seconds. Dow’s coatings and plastics division noted Eureka provided researchers additional time for creative brainstorming, laboratory experiments, and strategic R&D advancement, effectively revolutionizing the preliminary research phase and enabling faster innovation cycles.
Predictive Simulation for Material Feasibility and Performance
AI further enhances materials research by predicting feasibility and performance through advanced simulation models. These models accurately forecast how materials or chemical compounds behave under conditions such as extreme temperatures, mechanical stress, or corrosion. By virtually evaluating various options early in development, researchers swiftly eliminate unsuitable materials. AI simulations also support transitioning materials from lab-scale prototypes to full-scale production, preemptively identifying potential manufacturing issues, such as bottlenecks or material fatigue. This predictive approach helps companies mitigate costly late-stage development problems, improving efficiency and reducing overall project risks.
PatSnap Eureka AI Agent in Materials Science
PatSnap Eureka AI Agent offers a unique patent search mechanism specifically designed for materials science research. Traditional patent databases often fail to efficiently search by technical parameters, such as identifying patents mentioning polymers with specific tensile strengths or melting points. Eureka Materials employs a sophisticated Properties, Units, and Measurements (PUM) model, enabling users to query patents based on exact material-specific data. Materials engineers simply input desired properties or composition profiles, and Eureka swiftly retrieves relevant patents and documents matching these precise criteria. By automating patent scouting through material properties, Eureka significantly reduces the time researchers would otherwise spend manually reviewing documents, enabling faster, targeted innovation in developing novel materials.
Accelerating Material Performance Analysis
Another highly valued feature within PatSnap Eureka is Material Performance Analysis. This capability allows users to input specific parameters and quickly receive a detailed digest of relevant data and references. Dr. Emily Carter, a senior materials research scientist, highlights its transformative impact: “As a materials engineer, I used to spend hours searching for data. SPUM analysis changed that—just a few inputs deliver precise results quickly. It’s a true game-changer for saving time and driving innovation.” This feature dramatically shortens research cycles from hours to minutes, providing precise and actionable insights that researchers might otherwise overlook. By eliminating tedious data collection, Eureka empowers scientists to concentrate more on interpreting results and driving material innovation forward.
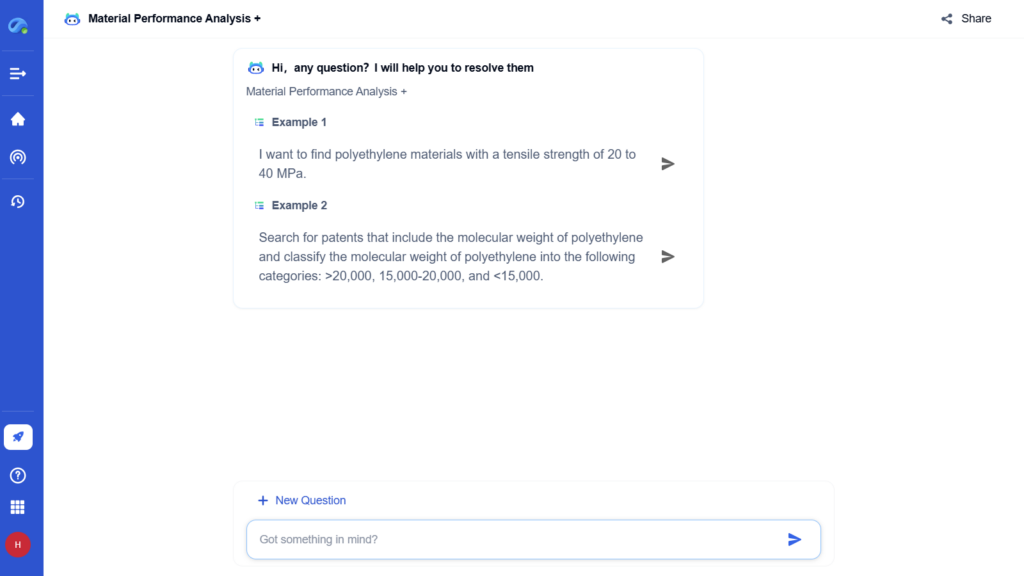
Integrating TRIZ Methodology for Inventive Problem Solving
PatSnap Eureka’s integration of the TRIZ (Theory of Inventive Problem Solving) methodology significantly boosts the problem-solving capabilities of materials scientists and engineers. Eureka’s “Find Solutions” agent applies TRIZ principles to address complex materials challenges, such as developing a lighter yet stronger composite for aerospace applications. Users have praised Eureka for significantly accelerating idea generation by suggesting innovative principles and analogies from diverse industries. For instance, it might identify techniques from semiconductor manufacturing applicable to polymer science, facilitating cross-domain innovation. Eureka effectively acts as an expert consultant available on-demand, bridging knowledge gaps across engineering disciplines.
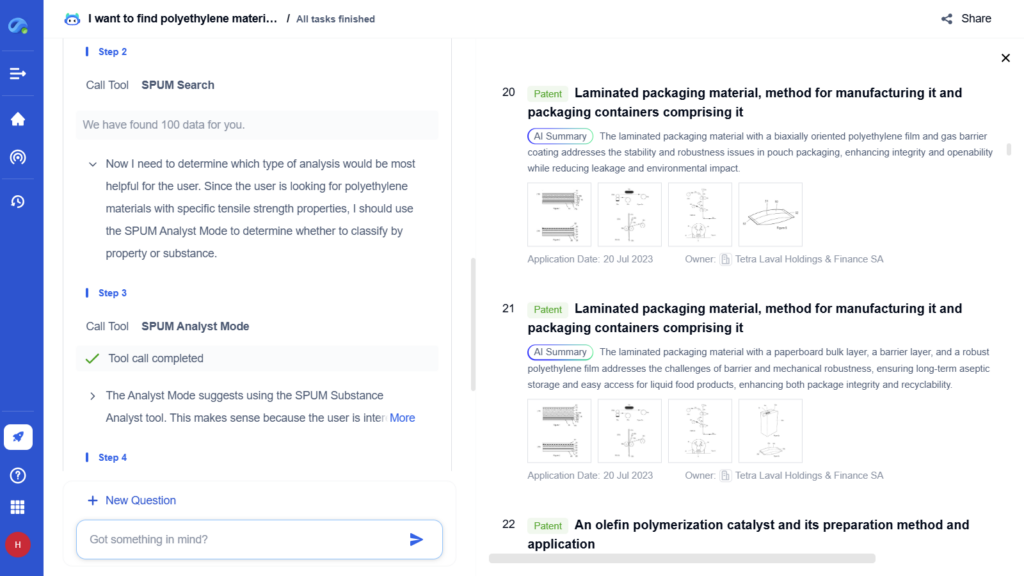
Ensuring High-Quality, Trustworthy Data
PatSnap Eureka’s effectiveness is underpinned by a comprehensive and rigorously maintained data pipeline. Eureka leverages PatSnap’s vast curated databases comprising material properties, scientific literature, patent filings, and market intelligence. This data, regularly updated and structured through robust ontologies, substantially enhances the AI’s output quality and reliability. Given that even minor oversights in materials R&D can derail projects, this data reliability is invaluable. PatSnap’s robust system handles over 2 billion data points across 20 specialized domains, significantly reducing errors and nonsensical AI responses. PatSnap reports a 60% reduction in inaccuracies compared to general-purpose AI, giving engineers greater confidence and efficiency by reducing the need for extensive fact-checking.
Real-World Impact: Enhanced Innovation and Efficiency
Eureka AI Agent demonstrably accelerates materials innovation cycles and improves decision-making. Organizations using Eureka report substantial efficiency improvements, including a 75% boost in intellectual property research productivity and a 25% reduction in overall R&D costs by eliminating redundant experiments and focusing resources strategically. Practical examples include materials manufacturers saving months of development time by quickly identifying viable solutions early in their R&D processes. Eureka also helps validate project feasibility by analyzing extensive data sets to identify potential issues or optimization opportunities. By serving as a reliable analytical partner, Eureka enables materials scientists to approach innovation with greater confidence, accelerating the journey from concept to market-ready materials. Ultimately, PatSnap Eureka is indispensable, not as a replacement for scientists, but as a powerful enhancement to their capabilities, ensuring quicker development and commercialization of groundbreaking materials.
Conclusion
Artificial intelligence is profoundly reshaping research methodologies and accelerating innovation cycles in both biological and materials sciences. Through detailed real-world applications and compelling case studies, we see AI’s transformative potential clearly demonstrated in the rapid identification of novel antibiotics, personalized medicine advancements, high-efficiency materials discovery, and streamlined experimental workflows.
The PatSnap Eureka AI Agent exemplifies this new era of intelligent research assistance, significantly enhancing productivity, reducing costs, and improving accuracy across life sciences and materials research. By automating complex data analysis, facilitating rapid insights, and offering cross-disciplinary innovation suggestions, Eureka empowers researchers to break new ground faster than ever before.
Ultimately, integrating advanced AI tools like PatSnap Eureka into scientific research not only accelerates discovery but also enables researchers to explore broader, more complex questions that were previously impractical. As AI continues to evolve, we can anticipate even more groundbreaking discoveries, faster commercialization of innovative solutions, and a deeper, more nuanced understanding of complex biological and materials systems. The future of scientific research is here, and it is unmistakably powered by artificial intelligence
To get detailed scientific explanations of AI for research, try Patsnap Eureka.
